Machine Learning (ML) is a powerful tool for researchers analyzing microscopy images in various fields. ML offers many benefits to the image analysis workflow, from easy segmentation to the detection of complex objects. Ultimately, ML-powered image analysis tools empower scientists to focus on insights.
Why use machine learning in image analysis?
While there are multiple benefits to ML, here are the top 3 benefits:
Easier workflow since users simply provide examples of the objects of interest instead of the rules that define the objects (size range, intensity threshold, etc)
The model can detect complex objects as it contains characteristics beyond common measurements
ML algorithms can adapt to experiment/imaging conditions changes by adding more examples
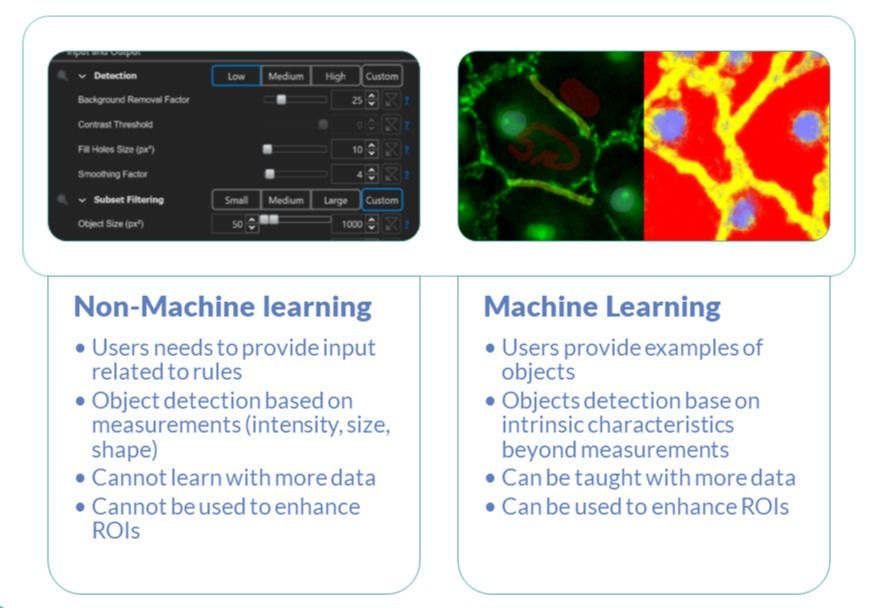
What can I accomplish with ML in image analysis?
Results from ML tools in microscopy image analysis can be grouped into 2 broad categories: image enhancement and object segmentation.
Image enhancements workflows typically create a confidence map that augment or separate your objects of interest from the background. The confidence map resembles a high signal-to-noise fluorescence image where pixel intensity is associated with the ML algorithms' confidence that this is your object of interest. High intensity values is associated with high confidence. Below are a few examples of the type of enhancement you can accomplish with ML:
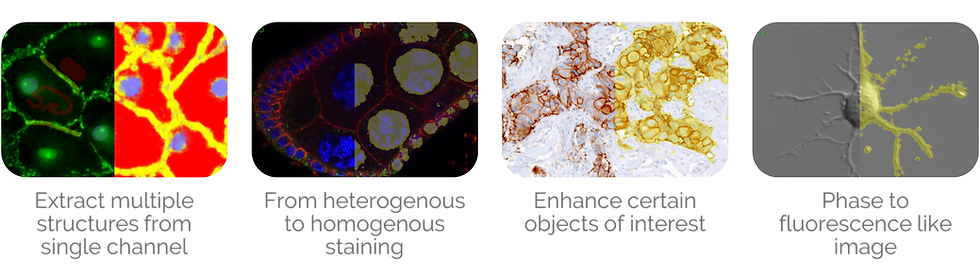
ML is also used to segment difficult to analyze objects such as cells in phase contrast images or unusually shaped cells. Once detected, you can obtain volume, average intensity, and many other measurements. Below are a few examples:
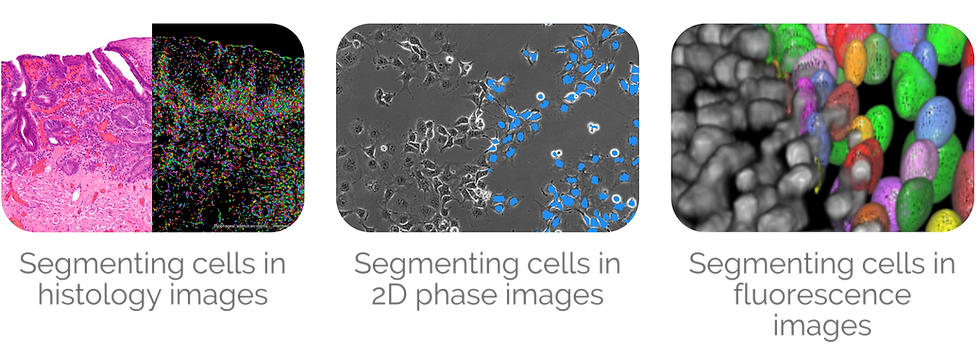
How do I create an effective ML-powered Pixel Classifier?
One of Aivia's most popular features, Pixel Classifier, is powered by machine learning algorithms containing the benefits and capabilities mentioned above. While there are many things to consider when creating an effective Pixel Classifier, here are the top 3 things to keep in mind:
Provide examples of the variety in your objects - small and large, bright and dim, round and funky-shaped objects - the more variations you capture, the better the algorithm will understand your objects
Preview, preview, preview - it's difficult to predict exactly how your examples will change the model so preview frequently to make sure the training is going in the right direction
Save your pixel training file - this will allow you to adjust the model if your project/imaging settings changes
Where can I find more information about Aivia's Pixel Classifier?
The examples shown above were created using Aivia's Pixel Classifier feature so if you are looking to learn more about ML-powered image analysis, here are a few resources:
Pixel Classifier FAQ: https://www.aivia-software.com/post/faq-pixel-classifier
Tutorial video for 3D smart segmentation: https://youtu.be/d4k5jgHLIDg
YouTube playlist of examples: https://www.youtube.com/playlist?list=PL4rtkW_e-B4wiX3EYRvvzbcLBm-c2yvok
Image credits for the images above:
Comments