Presentation time: Sunday Dec 8th at 1:30 PM
Poster number: P35/B36
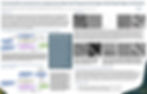
Abstract
Deep learning (DL) is emerging as a powerful tool that has attracted a lot of interest in microscopy image analysis. Using deep learning for automated 3D EM image boundary detection is a promising new development. However, this technology is currently only used by a small number of pioneering research groups. This is largely due to four major practical hurdles: (1) the requirement for multiple highly specialized and disjointed software libraries or tools to cover the entire DL train‐apply workflow; (2) the need to have extensive expertise to fine‐tune the training process; (3) the need to access high‐performance hardware to train DL networks, and (4) the difficulty and time‐consuming process of ground truth (GT) creation. Furthermore, the resulting deep models are specific to the trained experimental and imaging conditions (called “domain”) and are not readily applicable to images from other domains without significant re‐training. We are developing a novel deep learning powered app‐matching image conversion framework that converts images from a new domain to mimic the images from the domain where an application model (called “App”) is created and validated. Therefore, the validated App from a training domain can be applied to a new domain by converting new domain images to the training domain. This renders the App universally applicable without new domain specific re‐training. We validated the app‐matching image conversion framework in the 3D EM image boundary detection applications. A U‐net (the App) is trained to segment 3D neurites in EM images from the ISBI 2013 challenge (the training domain). The App is applied to new EM images acquired at the Rachel Wong Lab from the University of Washington (new domain). The new domain images are converted through app‐matching image conversion and then the App is applied. The results are close to a new U‐net specifically trained using the new domain data. Furthermore, we demonstrated that the app‐matching image converter can be trained with as few as a single image from the new domain. This represents a significant advantage in practical applications. We are working on application enhanced conversion and artifact rejection that should further improve the applicability and performance of our novel app‐matching image conversion framework.
Authors
H. Sasaki, W. Yu, R. O. Wong, L. A. G. Lucas, C. Huang, J. S. J. Lee
W. Yu and R. Wong are a part of the Department of Biological Structure at the University of Washington, Seattle, WA.
Other authors are part of DRVision Technologies LLC, Bellevue, WA